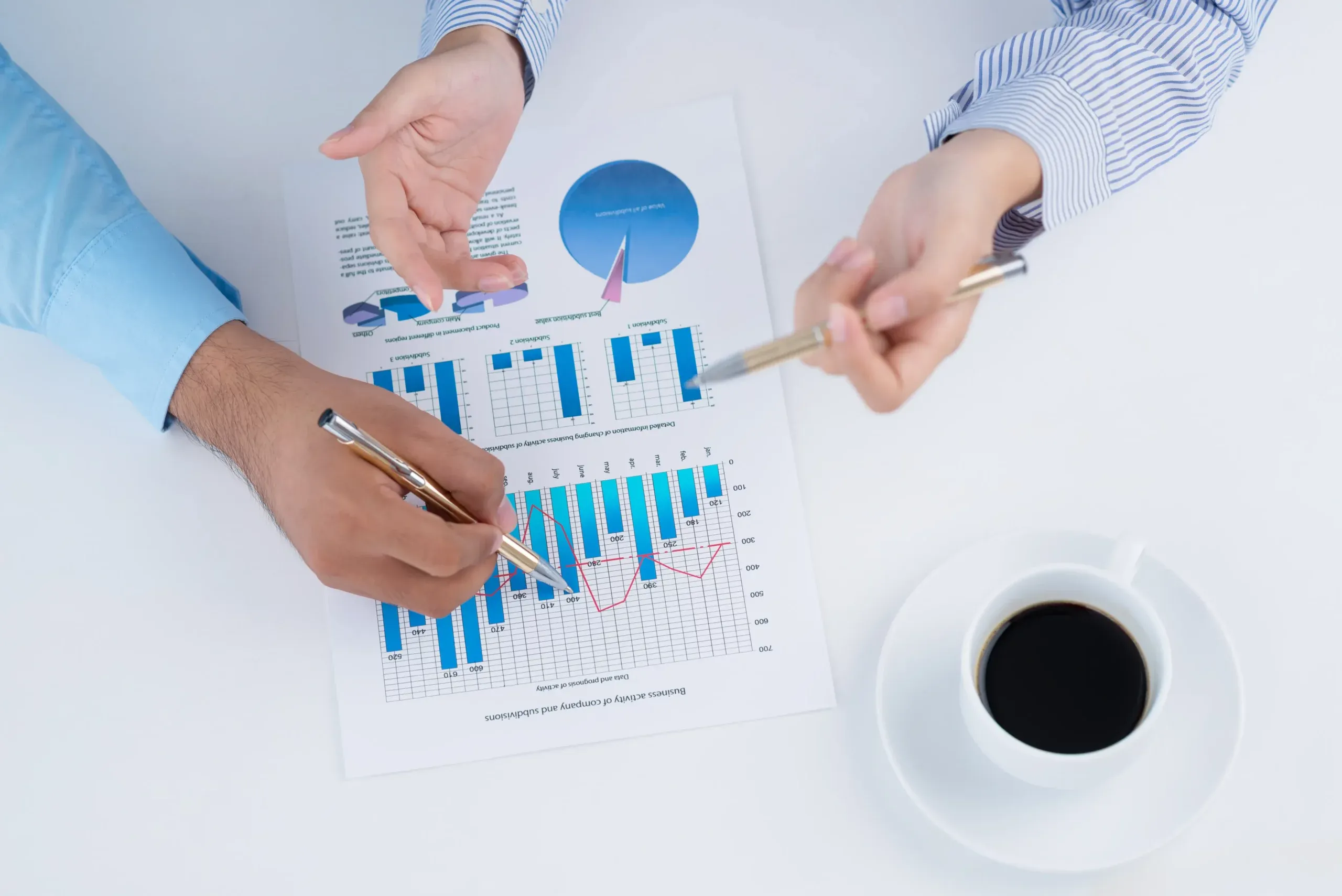
Leveraging Big Data for Strategic Financial Analysis
Introduction
In today’s digital age, data is abundant, and its analysis has become crucial for businesses to gain actionable insights. Big data analytics offers vast potential for strategic financial analysis, enabling organizations to make informed decisions, mitigate risks, and seize opportunities in a dynamic marketplace. In this comprehensive guide, we’ll delve into the significance of leveraging big data for strategic financial analysis, explore key methodologies and tools, and address common questions surrounding this transformative approach.
Why big data for financial analysis?
Data volume: Big data provides access to vast amounts of information, including transactional data, market trends, customer behavior, and more. Analyzing this data can uncover patterns and correlations that traditional methods may overlook. With the exponential growth of data generation, organizations can tap into diverse sources to gain comprehensive insights into financial performance and market dynamics.
Real-time insights: With big data analytics, financial analysts can access real-time data streams, allowing for agile decision-making and faster responses to market changes and emerging trends. Real-time data analytics empowers organizations to monitor key metrics, such as sales figures, market sentiment, and operational performance, enabling proactive adjustments to strategies and tactics.
Predictive analytics: Big data enables predictive modeling and forecasting, empowering organizations to anticipate future market movements, identify potential risks, and capitalize on opportunities proactively. By leveraging historical data, market trends, and external factors, predictive analytics algorithms can generate accurate forecasts, aiding in strategic planning, resource allocation, and risk management.
Competitive advantage: By harnessing big data analytics, businesses can gain a competitive edge by uncovering hidden opportunities, optimizing processes, and enhancing strategic planning and execution. With access to comprehensive data insights, organizations can identify market trends, consumer preferences, and competitive threats, enabling them to differentiate their offerings, improve customer experiences, and drive sustainable growth.
Methodologies and tools for big data financial analysis
Data collection and integration: The first step in leveraging big data for financial analysis involves collecting and integrating data from various sources, including internal databases, external market data providers, social media platforms, and IoT devices. Advanced data integration platforms and tools facilitate seamless data aggregation, cleansing, and normalization, ensuring consistency and accuracy across diverse data sets.
Data cleaning and preprocessing: Once data is collected, it undergoes cleaning and preprocessing to remove inconsistencies, errors, and duplicate entries. This ensures that the data is accurate and reliable for analysis. Data cleaning techniques, such as outlier detection, missing value imputation, and data standardization, enhance data quality and integrity, enabling meaningful insights and decision-making.
Descriptive analytics: Descriptive analytics involves summarizing historical data to gain insights into past trends, performance metrics, and key drivers affecting financial outcomes. Techniques such as data visualization, dashboards, and reporting tools aid in this process, providing stakeholders with intuitive visualizations and actionable insights. Descriptive analytics enables organizations to monitor key performance indicators (KPIs), track business metrics, and assess historical performance, facilitating data-driven decision-making and performance evaluation.
Diagnostic analytics: Diagnostic analytics focuses on understanding why certain events occurred by identifying correlations and causal relationships within the data. This helps financial analysts pinpoint factors influencing financial performance and identify areas for improvement. Root cause analysis, correlation analysis, and regression modeling are common diagnostic analytics techniques used to identify trends, anomalies, and patterns, enabling organizations to diagnose performance issues, assess risk factors, and optimize resource allocation.
Predictive analytics: Predictive analytics leverages statistical algorithms and machine learning techniques to forecast future trends, market movements, and financial outcomes. By analyzing historical data patterns, predictive models can anticipate potential risks and opportunities, enabling proactive decision-making. Time series analysis, regression analysis, and machine learning algorithms, such as neural networks and decision trees, enable organizations to develop predictive models that optimize forecasting accuracy, mitigate risks, and capitalize on market opportunities.
Prescriptive analytics: Prescriptive analytics goes beyond predicting future outcomes by recommending actions to optimize financial performance. By simulating various scenarios and evaluating the potential impact of different decisions, organizations can make data-driven choices that align with their strategic objectives. Optimization algorithms, simulation models, and decision support systems enable organizations to identify optimal strategies, resource allocations, and risk mitigation measures, enhancing decision-making effectiveness and strategic agility.
Faqs:
Q1: What are the primary challenges associated with leveraging big data for financial analysis? A1: Some of the main challenges include data quality issues, privacy concerns, data security risks, and the need for specialized skills and expertise in data analytics and financial modeling. Data quality management, privacy protection measures, and cybersecurity protocols are essential to address these challenges and ensure the integrity, confidentiality, and availability of financial data.
Q2: How can organizations ensure data privacy and security when analyzing sensitive financial data? A2: Implementing robust data governance policies, encryption techniques, access controls, and compliance measures can help safeguard sensitive financial information from unauthorized access and data breaches. By establishing data privacy frameworks, conducting regular security audits, and investing in secure data storage and transmission technologies, organizations can mitigate risks and ensure regulatory compliance.
Q3: What role does machine learning play in big data financial analysis? A3: Machine learning algorithms enable organizations to analyze large volumes of data quickly, identify patterns, and make accurate predictions, enhancing the accuracy and efficiency of financial analysis processes. Supervised learning, unsupervised learning, and reinforcement learning algorithms enable organizations to automate repetitive tasks, detect anomalies, and extract actionable insights from complex data sets, driving innovation and competitive advantage.
Q4: What are the key considerations for selecting the right big data analytics tools for financial analysis? A4: When choosing big data analytics tools for financial analysis, organizations should consider factors such as scalability, flexibility, ease of integration, advanced analytics capabilities, and total cost of ownership. Cloud-based platforms, open-source frameworks, and commercial analytics suites offer a wide range of features and functionalities tailored to diverse business needs and analytical requirements. Evaluating vendors, conducting proof-of-concept trials, and soliciting feedback from end-users can help organizations identify the most suitable tools that align with their strategic objectives and technical infrastructure.
Q5: How can organizations address data governance and regulatory compliance challenges when leveraging big data for financial analysis? A5: Data governance and regulatory compliance are critical considerations for organizations leveraging big data for financial analysis, particularly in highly regulated industries such as banking, healthcare, and finance. Implementing robust data governance frameworks, data quality management processes, and compliance controls ensures the accuracy, integrity, and security of financial data while adhering to industry regulations such as GDPR, HIPAA, SOX, and Basel III. Collaborating with legal, compliance, and cybersecurity experts, conducting regular audits, and staying abreast of regulatory updates and best practices help organizations mitigate risks and maintain regulatory compliance throughout the data lifecycle.
Conclusion
In conclusion, leveraging big data for strategic financial analysis offers significant opportunities for organizations to gain insights, mitigate risks, and drive competitive advantage in today’s fast-paced business environment. By harnessing advanced analytics techniques and tools, businesses can unlock the full potential of their data assets, enabling informed decision-making and sustainable growth. Embracing big data analytics is not just a trend but a strategic imperative for organizations seeking to thrive in the digital era. With the right methodologies, tools, and expertise, organizations can harness the power of big data to transform financial analysis, optimize performance, and achieve their strategic objectives.