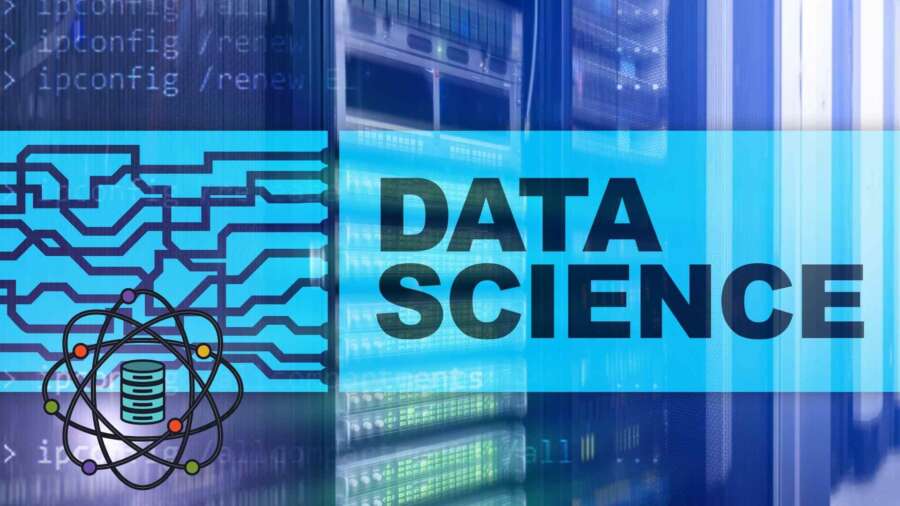
Imam Hoque, CPO and Co-Founder at Quantexa,
Despite an over two decade fight and billions of dollars spent on technology and processes , money laundering still happens and while some of it is detected, much goes unnoticed as criminals continue to find new and innovative ways to launder money. As technology advances, criminals have become more sophisticated at disguising income, taking advantage of faster payments, capital markets, and open banking. Unfortunately, the AML systems at banks’ disposal haven’t been sufficiently advanced until very recently. With giant steps in data analytics technologies, how can the financial sector now overhaul its processes to push back against increasingly innovative criminals?
A flawed system
For Anti Money Laundering (AML) compliance, it’s far more useful to know who is making the transaction than how much money is being moved. Unfortunately, most AML systems are rules-based, flagging alerts based only on the value, volume or statistical unusualness of the transaction – without the context needed to see whether the transaction is legitimate or suspicious. This limited view of data/activity has caused the false positive plague that currently burdens the industry and also allowed money laundering activity to go undetected by hiding in plain sight.
The current traditional approach complicates the monitoring process by introducing a range of overly simplistic solutions that can’t spot new patterns or behaviours, and can only respond to specific triggers, or ‘rules’: for example, any transaction to a personal account over £50,000 will get marked as suspicious. If the criminal understands this limit and now reduces the amount to £35,000 it passes through the mousetrap. If the bank reduces the threshold then they get many more alerts, and more than likely the criminal will reduce the amount again. With such narrow behavioural parameters, ordinary, normal activities often get flagged as potentially unusual . For instance, if an individual makes a number of purchases in quick succession, or deposits large amounts of money at ATMs. Taken on their own, these by no means indicate the person is committing a financial crime, but will get flagged in the systems as a potential risk.
Being ineffective at separating innocent behaviours from financial crime threats, this rules-based approach creates a surprising ratio of false positives: 90 to 95%, an unsustainable proportion that taxes human teams in charge of overseeing the alerts. These analysts’ skills are wasted in investigating Level 1 alerts, of which 9 out of ten are false alarms anyway. They need technology to refine the alerts emitted, in order to free their hands for the more sophisticated, top-level investigations.
What’s missing? Context
Ultimately, it’s the meaning behind data that matters most when it comes to detecting and stopping financial crime. This meaning is the “context”, which reveals what the data means in the real world.
Context provides a broader understanding of an event, person, or item, framing knowledge in a larger picture and giving it perspective.
For instance, you wouldn’t make a decision to buy a house after only looking through the front windows. Unfortunately, this is what happens when AML systems look at transactions in isolation.
Here is an example of how context helps determine suspicious vs. innocent activity: Any individual can receive a high value payment. The question is where did that money come from? Did it come from their employer and have they just received a large bonus? or did it come from a solicitor that would indicate a property sales or inheritance? However what would happen if this money came from a tax haven country or it was paid in by an organisation that has been known to be a front for Terrorist Financing and links to extremism.
A better approach to detecting financial crime
To succeed, banks and financial organisations need to put data at the centre. Using an approach that brings together internal, external, publicly available and transactional data, banks can create a complete and full picture of their customers, their customer’s customers and any connection between and beyond them to make more informed decisions on whether something looks suspicious, in significantly less time. This is exactly what AML investigators do manually today.
When this full picture of activity is automatically created by software and then used in the detection and analytical processes, it effectively combines human intelligence with artificial intelligence (AI). This makes it much easier to detect activity, reduce false positives, and more quickly escalate the number of real incidents that are captured.
AI can pour through more data faster than its human counterparts, making it possible for banks to find out more about criminal networks than ever before. Transactions leave a data footprint, and analytics can start to paint a picture of what criminals are up to – the ultimate goal to be able to map this activity in near real-time.
Contextual Decision Intelligence
This approach can be summarised by the term Contextual Decision Intelligence (CDI)- a method that combines data context with powerful artificial intelligence capabilities.
CDI starts with dynamic entity resolution, the process by which the billions of data points spread across multiple systems become connected into a trusted, accurate single view. This offers a complete, meaningful view of data across the enterprise that reflects real-world people, places, and organizations—and the relationships between them. It also enhanced the internal view of data with connections to external data sources providing an increase in valuable and relevant data on external relationships for example.
Entity resolution helps businesses make sense of their data by working out whether multiple records are referencing the same real-world entity, such as a person, organisation, address, phone number, bank account, or device. This is key for AML compliance. To do this, algorithms analyse disparate data points and resolve them into a single, unique, trusted entity.
Creating the network
Once they have improved data quality, network generation creates a dynamic view of relevant connections, entities, and data for a specific decision, helping uncover connections and behaviours that are impossible to see when looking at data in isolation. Criminals use companies to hide their identities, it is only through networks that hidden risks of corruption, tax avoidance and sanctions can be uncovered. From there, advanced analytics models can be built that allow businesses to make data-driven decisions. =
Relationships, not rules
The world is a series of complex relationships, so why not model that information to detect financial crime? Chasing criminals based on a loose, untargeted set of behavioural parameters will never provide long-term, sustainable results, no matter how much money is invested.
However, through a combination of smart data science, cutting-edge algorithms, and the right technology, financial institutions can transition away from simply monitoring and investigating, and instead put themselves at the heart of disrupting financial crime.